Sentiments Analysis
“What if technologies could help us improve conversations online?” is a question raised by the tech giant Google’s Counter Abuse Technology (CAT) team, which resulted in the birth of the PerspectiveAPI project. Although still at its early phases of development, the project is providing new tools for identifying whether an online comment could be perceived as toxic to a discussion. The project is a partnership between Google, Wikipedia, The New York Times, The Economist and The Guardian.
Computer scientists have shown increasingly wide interest in studying human emotions and how they are linked to social issues, through what is being referred to as Sentiment Analysis. Examining emotions such as sadness, anger and personal preferences under empirical conditions not only helps marketers understand people and point their promotional campaigns in the right direction, it allows for a possible justification of practices and ideologies behind the behavior being analyzed, which can lead to a deeper understanding.
Psychologists and social scientists were doing this ever since their disciplines began: studying behaviors and analyzing communities. But when computer scientists intervene, a new angle is presented on the table. In a research team where the three work together, taking advantage of the rapidly advancing software and hardware technologies, complexities of the examined phenomena are examined in more efficient manners.
Examining Hate Speech
Taking hate speech for example, it is indeed a complicated issue. Hate is subjective and interpreted differently by people of various backgrounds and origins. Relying on the context, it is expressed in multiple explicit and implicit forms. Moreover, the richness of a certain language or dialect might make it difficult to identify hate speech.
Online and through digital platforms, hate speech perpetuates quickly. Many groups on social media are driven by various factors such as religion, ethnicity, adopted political and moral ideologies and many more. Coming across hateful, racist or sexist statements on the comment section becomes the norm after navigating social media spheres for a while. The huge number of online posts perpetuating hate speech calls for the intervention of more sophisticated computational linguistic analysis using techniques developed for “Big Data”.
Sometimes, it is harder to automate detection of hate speech within a huge mass of messily written posts on social media. Other times, it is more challenging to identify interesting associations between hate speech and other factors under the conventional settings. Time and resources can be limiting as well, since automating would take a long time to design and build, and it won’t serve the final goals in their entirety. That’s why researchers engage in collaborations with computer scientists within a sub-field of computer science called NLP, or Natural Language Processing. Through NLP, huge texts of posts or documents of written format are analyzed with the help of mathematical models. The job of these models is mapping inputs (words, sentences) with output (decision: toxic comment or not) with the help of mathematical formulas.
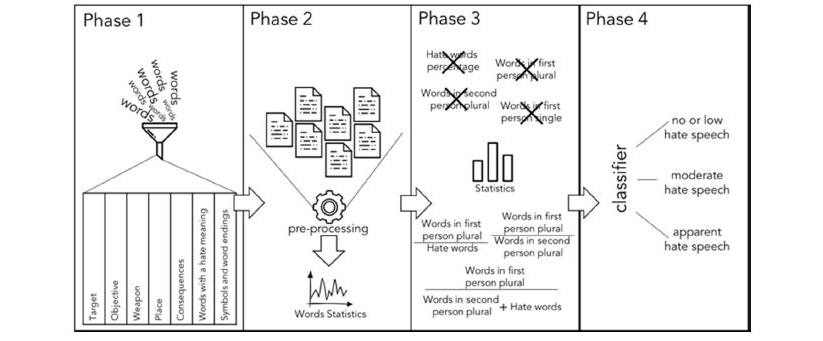
An algorithmic classification processes of text analysis. Resource: Detecting of hate speech within the terrorist argument – Hellenic Air Force Academy, Greece.
Online Hate Lecixons
The online hate speech research journey starts with extracting data to begin the analysis. And what are better resources for that than Twitter, and the comments sections on Facebook and YouTube? As these websites provide access through their APIs, or Application Platform Interface, extracting any relevant data is simpler with search-like features that help with collecting and storing texts in the format the user specifies. The data extracted from Twitter -or any other website that allows for this kind of extracting- comes in a textual form of the tweets being posted, along with other meta-data such as: location, device used for tweeting, time, language, and more. This gathered data helps researchers in constructing “hate dictionaries” or lexicons that contribute to automating the process of online hate speech detection. These dictionaries and datasets are composed of keywords that are more frequently used within hate speech, and can later be used to automate the process of identifying toxic and hateful comments.
An example of such dictionaries that can help automating the identification of online hate was developed by PeaceTech Lab Africa, a non-profit based in Kenya. They developed the online hate lexicon of hate speech terms used by South Sudanese communities on social media between August and November 2017. The Lab recently published another lexicon with the same focus in Cameroon. Another example is the hate-words lists developed by researchers from Qatar Computing Research Institute and Hamad Bin Khalifa University, published on Github.
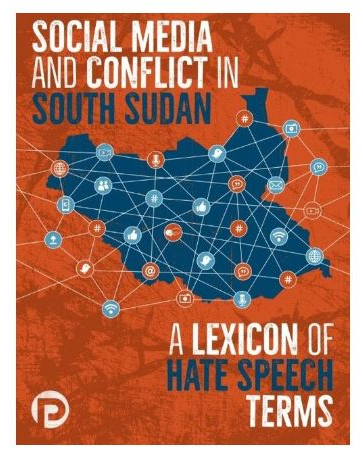
Source: PeaceTech Lab.
In other more exploratory research on hate speech, some algorithmic models that help in automatic identification of hate are specialized in pointing out associations and linkages between different factors, and are able to identify relationships that enable us to answer questions such as: which countries are more sensitive to hate? And which are less?
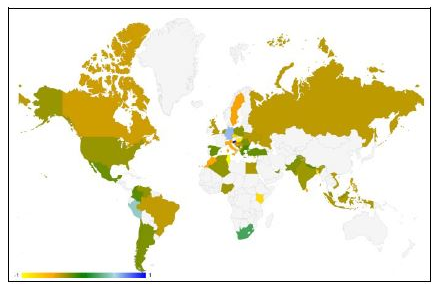
Normalized hate interpretation score at the country level. Resource: A statistical analysis of hate using crowdsourcing ratings – Qatar Computing Researching Institute, Hamad Bin Khalifa University.
As complicated at it seems to socially analyze messy and diverse hate speech texts with computational means, the answers that this approach helps to unfold are encouraging. Deep social network analysis and the interpretations it brings can help present a better understanding of motives, which groups are targeted the most, how people interact with hate speech online, and detect more hidden types of offensive language.
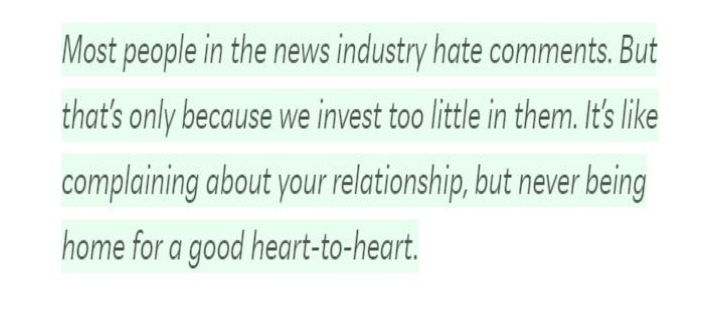
Source: Medium.com: Help us shape the future of comments on The Economist.
Nevertheless, raising awareness and training on the responsible use of social media in general and online-comments section in particular would pave the way for a more peaceful digital environment. In their efforts to address how online hate can contribute to the offline conflict, the #defyhatenow initiative has worked on a number of campaigns, both online and offline, to tackle the problem by first raising awareness. Examples of these are the Social Media #PeaceJam campaigns held yearly on the UN World Peace Day, cultural and sporting events, street theatre, #DEFY the film, #Peace4ALL and #ThinkB4Uclick song & campaigns.
In addition to films, materials and training workshops on countering online hate, the #defyhatenow blog highlighted South Sudanese singers, poets, and professional women sharing their peace-building activities & stories that inspire.
Final Thoughts
Comment sections are where many insights and intriguing conversations start. We don’t want them switched off because of trolls and offensive language, and we can’t simply and easily prevent people from spreading hate across social media. Maybe what we really need is a closer inspection of the problem, by paying more attention to the toxic and hateful language online. The complexity and dimensionality of online hate speech calls for new ways of thinking through developing innovative technological & social solutions combating hate speech.
At the end, we praise the technologies that give us the chance to do so.
Resources:
- Salminen, J., Veronesi, F., Almerekhi, H., Jung, S.G. and Jansen, B.J., 2018, October. Online Hate Interpretation Varies by Country, But More by Individual: A Statistical Analysis Using Crowdsourced Ratings. In 2018 Fifth International Conference on Social Networks Analysis, Management and Security (SNAMS) (pp. 88-94). IEEE.
- Lekea, I.K. and Karampelas, P., 2018, August. Detecting Hate Speech Within the Terrorist Argument: A Greek Case. In 2018 IEEE/ACM International Conference on Advances in Social Networks Analysis and Mining (ASONAM) (pp. 1084-1091). IEEE.
- Wikipedia – Research: Detox.
- The Economist: Help us shape the future of comments on economist.com.
- The Guardian: The web we want.
- New York Times partnering with Jigsaw to expand comments capabilities.